As a UX Consultant at DB Systel’s dx.house, I had the opportunity to participate in a training by Datentreiber on their Data & AI Business Design method. Thanks to a project led by Gaetano Rizzo, I was able to test and apply this method in practice. The project offered an excellent opportunity to explore how the structured approach of Data & AI Business Design, combined with Datentreiber’s freely available tools, can be used to design data-driven products that align user needs with business objectives. This experience was invaluable in discovering new ways to connect analytics, user-centricity, and business value.
User Understanding as a Central Pillar
User understanding is the cornerstone of designing impactful data products. It begins with identifying the users and gaining a deep understanding of their workflows, goals, and pain points. Through targeted methods like interviews and observations, we collect insights that help align the design of data-driven solutions with user needs. This ensures that the product delivers real value by solving tangible problems and improving user workflows.
This focus on user understanding also bridges the gap between technology and practical application. By integrating user feedback into the design process, we create solutions that are both intuitive and effective. Many projects fail due to low acceptance, often caused by overlooking the user perspective. A user-centric approach ensures not only higher adoption rates but also the successful alignment of data products with broader organizational objectives.
Business and Process Analysis
At the outset, we conducted a business and process analysis to establish the current state. This involved visually mapping out the business processes in detail (e.g., an application process with clearly defined start and end points). We then identified existing use cases and those already implemented.
A concrete example: For a process aimed at improving application handling efficiency, we outlined the workflow, highlighting key decision-making steps and involved stakeholders. The goal was to identify areas for improvement. A thorough assessment of the current state, including all existing applications, also provided insights into the organization’s AI maturity level.
User-Centered Focus
In the next phase, the focus shifted to the user:
We began by selecting the next-best use case and identifying the potential user. Through targeted interviews, we gathered detailed information about the user’s workflows, goals, and challenges. This user understanding is instrumental in crafting an analytical solution from the user’s perspective and delivering added value. Many analytical projects fail due to low user acceptance or minimal value creation because they are developed without considering the user.
To ensure a use case can be successfully implemented, it is essential to assess the technical prerequisites of the product or service. During the analysis of the data landscape, existing data sources were reviewed to uncover all relevant datasets. These sources included project lists and other documents. Finally, the data and information were consolidated to develop a technical-analytical concept.
Iterative Process and Outcomes
The Data & AI Business Design method proved to be highly effective in our workshops, particularly due to its strong emphasis on user understanding. This approach allowed us to develop a technical-analytical concept for a product or service. By following an iterative process, we delivered valuable insights into business processes, user needs, and data strategy, creating a solid foundation for an AI product.
I’m excited to continue refining this method and applying it to future projects.
About the author
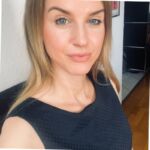
Madlen Kirsten (Senior User Experience Designerin || DB Enterprise Applications || Human-Centered-AI at DB Systel GmbH)
Don’t miss any more blog posts, subscribe to our newsletter.